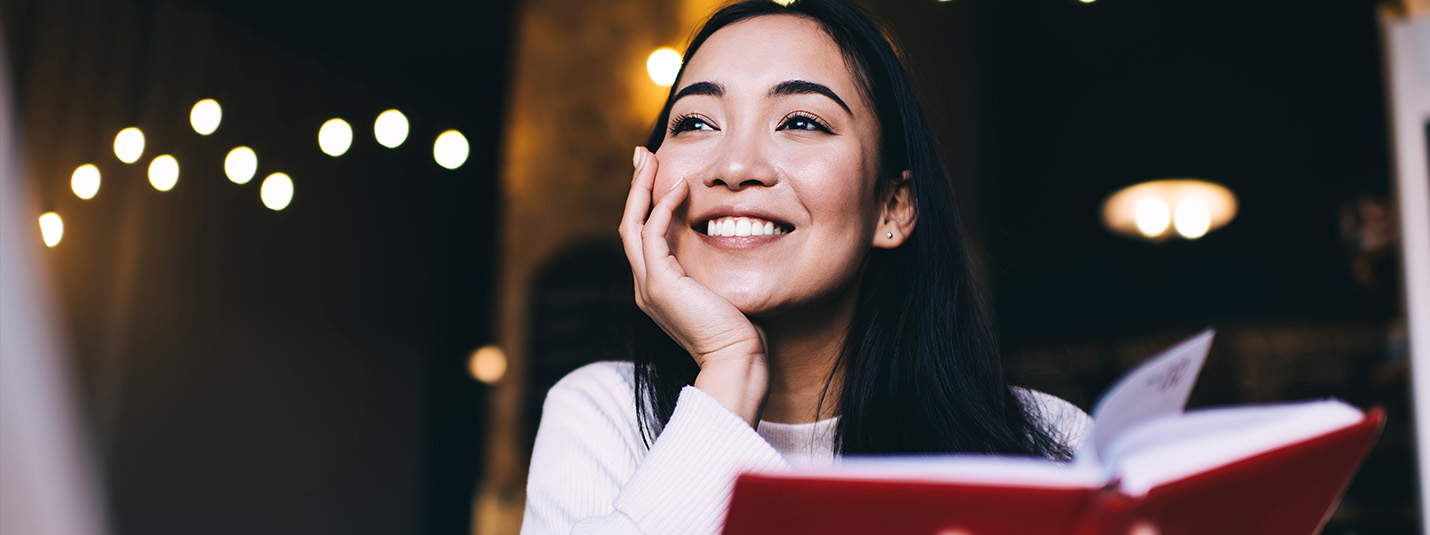
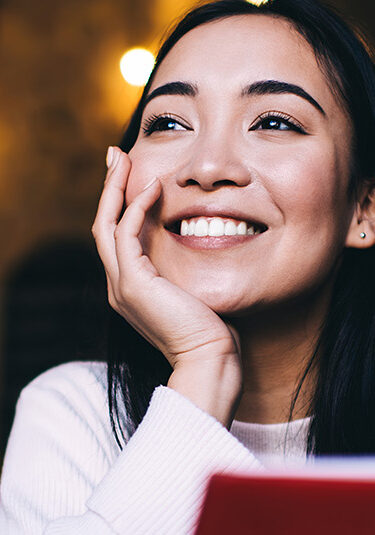
News
Q-Free published in Tolltrans magazine: a new view on video-based tolling
A primary cost driver of any video toll collection system is the ongoing operational expense (OPEX) caused when trying to convert video transaction imagery into plate reads suitable for billing toll road patrons. A clear understanding of the processes that make up any image review system and the performance factors that determine its overall OPEX is essential in order to accurately compare the true cost between different image review system suppliers, to assess the potential cost savings of any contemplated modifications to an existing system, or to define requests for proposal requirements that truly minimize a toll agency’s image review OPEX.
It is no secret that image review performance and OPEX are highly dependent upon the input: image quality. Poor image quality increases OPEX since fewer plate images can’t be automatically read, more images require time-consuming manual enhancement and, generally, more review, time is needed per transaction to discern unclear character components. In addition to an increase in OPEX, poor image quality also leads to a higher probability of system read errors and leakage due to a general increase in confusion between similar-looking plate characters.
Image Quality
So how does one define image quality in such a way as to maximize performance of an optical character recognition (OCR) engine and mitigate increases in OPEX? Currently, most toll agencies define image quality only in terms of human readability. While human readability is a necessary condition for image review, it is not sufficient by itself to minimize OPEX. Image quality must be better than that which humans require in order to minimize OPEX.
The difference in image quality required for OCR engines is commonly exploited by websites. CAPTCHA (Completely Automated Public Tests to tell Computers and Humans Apart) images feature somewhat distorted, marginally resolved characters with weak contrast against interfering and/or noisy backgrounds. They are used to determine the presence of a human reader as distinct from an internet robot on a phishing expedition, and are successful because no known computerized techniques exist to accurately read all the characters in the images presented. License plate images can suffer from many of the same quality issues exhibited in CAPTCHAs and when this happens no OCR algorithm can possibly read them with high accuracy. The result is poor automation performance and much higher OPEX, even though the images meet the human-readable requirement.
Image quality must therefore be specifically defined to facilitate maximized OCR performance. Precisely defining image quality requirements entails that plate images be in sharp focus, have a sufficient amount of resolution on the characters (which cannot happen if the images are compressed too much), and the characters must have sufficient contrast with their immediate backgrounds.
Once image quality is addressed, the next critically important system element to consider is the OCR engine and the accuracy that agencies should demand from their image review system suppliers. To minimize OPEX, it is best to design and tune the OCR component to deliver the highest possible read rate at an accuracy rate very near to the desired final system accuracy rate (or its inverse: error rate). In North America, error rates of 0.05% (or accuracy rates of 99.95%) at 0.1% false reject rates have been achieved operationally at several of Q-Free’s clients. In Asia and in Europe, Q-Free has achieved plate read accuracy rates as high as 99.99% (0.01% error).
This, the error rate, is measured as the overall percentage of input transactions that the image review system outputs with an incorrect read despite all the automated and manual review processing employed by the image review”” system to try and achieve accurate answers. Error rate, therefore, includes all sources of image review system error, not just those from a particular OCR component within the transaction review process. The false reject rate is the overall percentage of input transactions that the image review system rejected as being unbillable even though the transaction images captured at the toll lanes contained all the information needed to obtain a suitable plate read.
Intrada System
Q-Free’s toll road image processor solution, lntrada Insight, takes a significant step forward for video-based tolling in North America in particular. lntrada Insight represents a fundamental shift in the approach to how image-based systems operate and combines improved performance through increased automation rates with high accuracy rates. This ensures that OPEX and leakage are minimized and revenues increased.
lntrada Insight is in fact a system of systems. lt brings together: Q-Free’s well established core technologies, including automatic license plate recognition (ALPR) powered by lntrada, OCR and vehicle fingerprinting; a data processing and warehousing component, which handles both long- and short-term storage and transaction automation, as well as improving user-friendliness by offering reporting capabilities; and a manual image review (MIR) subsystem with highly optimized user interfaces.
Intrada Insight also provides runtime monitoring of image quality, which delivers valuable feedback on the camera systems capturing the images at the roadside. The result is a solution that offers very high rates of automated image processing and reduces the cost burden associated with human interventions for manual processing. Intrada Insight has been proven operationally in the North American marketplace, in a variety of environments with a diverse array of plate types/styles, and the results are game changing. Intrada Insight has yielded accuracies that no other image review system provider is able to match and those large agencies that have taken advantage of its features have reaped the rewards, garnering OPEX savings in the millions of dollars per year range.
Traditional image review systems focus mostly on automation rates and manual review cost, paying little attention to accuracy. Most systems in North America achieve nominal accuracy rates in the 95-98% range. The Q-Free methodology shows that sacrificing even as much as 10-15% in automation rate, but reducing the error rate substantially, can save an agency millions of dollars. There are residual benefits to adopting the Q-Free approach, including the mitigation of expenses created by the mailing of erroneous toll bills or violation notices. The public relations headaches and time spent in front of state legislators or news reporters having to explain these errors are reduced substantially.
It is true that this approach differs from almost every other image review solution approach in the industry today. The current providers are only living up to the requirements being set by agencies and their consultants. This thinking, perpetuated by decades of pursuing higher and higher automation rates, has unfortunately come at the expense of accuracy, which in turn is costing agencies money.
Being accurate pays huge dividends and it is time to start expecting the right performance from image review systems.